In a rapidly evolving world driven by innovation like machine learning (ML), businesses cannot afford to be left behind. Artificial intelligence (AI) in general, and large language models in particular, are causing the biggest shift in business practices since the advent of the internet.
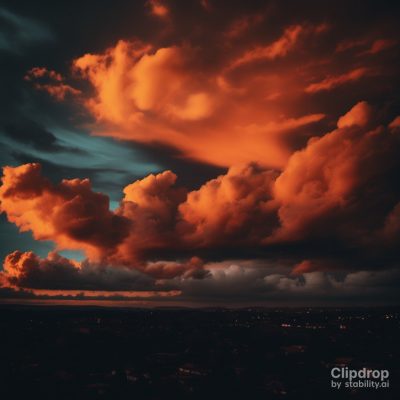
An AI generated image
- CEOs risk business obsolescence unless they act now. McKinsey recently articulated their view that generative AI’s impact on productivity could add the equivalent of $2.6 trillion to $4.4 trillion annually. According to McKinsey, significant value will be realized in customer operations, marketing and sales, software engineering, and R&D. Although the impact will be across all industry sectors, a few examples include $200 billion to $340 billion annually in banking and $400 billion to $660 billion a year in retail and consumer packaged goods. Further, generative AI and other technologies have the potential to automate work activities that absorb 60 to 70 percent of employees’ time today.
- Acting without care could create a nightmare scenario. Every CEO’s and CIO’s worst nightmare is waking up to news that their business has been hacked. It is even worse if the risk was preventable. Some business leaders might feel immune from this risk, but even tech companies like Microsoft suffer high-profile hacks. If the providers of the infrastructure that many of us rely on can be hacked, perhaps we should all be a bit more worried?Risks to security increase in periods of rapid technological change. The number of high-profile data breaches in 2023 is a stark reminder of these risks, with nearly half a billion breached records leaked so far this year! According to the MIT Technology Review, “CIOs would be reckless to adopt AI tools without managing their risks… [like] privacy and security breaches.” It follows that conventional security measures, like relying on chosen cloud or on-prem private networks alone, are likely not enough. Most concerningly, large language models (LLMs) use means that organizations are actively exposing data unwittingly. By using LLMs, businesses not only expose data and intellectual property but breach existing and future regulation.
- Regulation is on the horizon. LLMs use data that is input by many users to further train its model for future users. An employee could input data that contains confidential information, such as trade secrets, medical records, or financial data. If personally identifiable information is input, that is likely a breach of GDPR regulations. Users of LLMs need to further consider overarching GDPR principles like data minimization and fairness and the rights of data subjects, including the right to access and delete their data.These risks are being scrutinized under the European Union (EU) Artificial Intelligence Act. Recent developments like LLMs have caused the European Parliament to reassess “high-risk” use cases and how to implement proper safeguards. This includes a requirement for “use of state-of-the-art security and privacy-preserving measures”.
- How can organizations do ML well? Safeguarding LLMs will take a mix of different techniques 1) proper procedures, 2) active guardrails and 3) secure and private LLM environments. encloud focuses on enabling the third limb in combination with the second. How? encloud is about to launch its new private compute and data access tooling that works in combination with existing encryption and key management tools to ensure complete data privacy and security across the lifecycle. The flagship use case for our new launch is to enable confidential ML to ensure businesses can benefit from the potential of generative AI with minimal risk. The private compute tool leverages trusted execution environments, specialized chips that act like a vault for your data. In this vault, sensitive data can be combined with LLM models ensuring that the inputs, outputs, and the adapted model itself can be retained by the data owner.
Watch this space for further announcements about the launch and reach out to us at contact@encloud.tech to learn more!